Pineapples, design, league tables and clinical trials
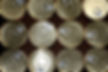
Few years ago, during my Ph.D. studies, I had the luck to be taught a statistics course by Stephen Senn.
Stephen, on top of being a skier and a hiker, is a brilliant teacher and presenter. He often includes in his talks stories and jokes whose meaning can be difficult to grasp at first for his students, but that become more and more revealing once one learns more about statistics and re-thinks about them. One of the jokes he told us that day, perhaps the easiest to understand even for beginners, was the following (slightly adapted from here):
Three scientists, a chemist a physicist and a statistician, are shipwrecked on a desert island with only a can of pineapple between them. They debate how to open it. Chemist: Put it in saltwater and wait for the can to corrode Physicist: Don't be ridiculous. That will take too long and the salt will ruin the flavour. Chemist (Aggrieved): So what's your solution? Physicist: Put it on a fire & wait for the pressure to build. The can explodes and voilà. You have pineapple. Statistician: Don't be silly. The pineapple will be spread all over the beach and mixed with sand. Physicist (Aggrieved): So can you help? Statistician: I could have done but you should have consulted me when you designed the can, and then I would have put a lid on it.
The most common misconception about statistics is that it is this magic discipline that, feeded with random data, returns spectacular charts and graphs (that generally selectively confirm one's hypotheses). In fact, I lost the count of times I have been asked to "do something with my data" or "perform some test to show that X is Y". But statistics is not the art of magically opening lidless data cans. It is the art of designing those cans with a lid, in order to make it possible to open them in the most simple and effective way.
Because of professional bias, I tend to reduce every problem to examples I am used to work with. I'd be tempted then to explain the importance of good design for statistical experiments using randomised controlled trials. I am not going to do that, though. Not directly, at least. I am going to talk first about a topic more people are possibly familiar with: league tables in sports.
Design and analysis
Let's focus on a specific sport: football, aka soccer. Organising a football league, or cup, is inherently an experiment to answer a statistical question. The experiment is generally made up of a series of 1-to-1 comparisons (matches).
The question is: which is the strongest team?
Finally the statistical bit comes from the fact that match results are subject to random variation around an expectation, as betters perfectly know: they can be seen as draws from a random distribution.
All statistical experiments are made up of at least 3 important parts: the design, the conduct and the analysis.
- The analysis is the bit that generally gets all the spotlight. It is creating the league table, fitting a model, doing a statistical test, etc etc. - Of course, there would be no analysis if it wasn't for a previous good study conduct: there is no league table until you have played football matches, no model or test until you have collected the data.
- But before that, there is the design: which matches should we play to maximise the probability to make the best team win the league? How should we plan our experiment and/or data collection to have the best chance to answer our research question correctly?
Let's assume we wanted to design a league with 16 teams. We have, broadly, two main ways of organising it: the European way and the American way. Being European I am much more familiar with our method, so my depiction of the American one might be very vague and inaccurate, but for the purpose of my post this won't matter:
- Round Robin: this is the European style. Each team will face each opponent precisely the same number of times. This is generally once, if playing on neutral ground, or twice, on a home-away basis. This is the design that achieves perfect balance, though of course teams' shape and condition can vary thoughout the season, there can be injuries, disqualifications, etc and this will surely affect the results.
- Regular season: let's just play as many games as possible, mainly, but not only, with teams closer geographically. At the end of the year the team who will be on top will be the best, even though maybe they will have played more home games against the strongest opponent, or more games in general against the weaker teams closer geographically. In American sports this often does not matter too much, because the main goal of the regular season (and the reason why it is called this way) is to set the grid for the play-offs, rather than to declare the winner.
Now let's compare with simulations these two approaches. We will use very simple models, just to show a simple point, so bear with me if these were not as realistic as possible! Let's draw the results of each match from a trinomial distribution (1-X-2), where the strongest team in the league has a probability of winning a home match that varies linearly from 40% against the second best team to 95% against the worst. For away matches let's vary this from 30% to 90%. As simulations only give approximate answers, subject to random variation, we will use a very large number of simulations (100 000) to make the residual random variability (what is known as Monte-Carlo Standard Error) as small as possible. Here every simulation is a full 30-match-per-team league. For the Round Robin scenario, we simulate one and exactly one match for each team against each opponent at home, and one and exactly one away match. For the Regular Season scenario instead, we simply draw each matchday schedule at random, without forcing any balance. So, potentially, team 1 could face team 16 at home for the whole season, though the probability of this happening would be as low as 1 in [Number with 36 zeros].
Running these simulations leads to the following results: 55.5% of leagues are won by the strongest team when designing the competition as a Round Robin, compared to 53% only when designing it "the American way". The difference is not that huge, but it still means that out of 100 championships, 3 more will be won by the strongest team using the balanced calendar.
Balance vs impartiality
There are, broadly speaking, two components in the design of a statistical experiment: structure and size. What we discussed so far is the structure: how should we choose how to generate the calendar? And how do we decide how many games should be played home and away? In clinical research, this would translate into questions like "how should we decide who to treat with a novel treatment or not?", "should we randomise?". The parallel between these two examples is stronger than one might think at first sight. Remember: a simple randomised experiment is one in which one of two (or more) treatment strategies or conditions is assigned to a group of participants completely at random, for example tossing a coin, or throwing a die. It is often said that the reason for randomising is to achieve balance between the two groups.What do we mean by balance? That the two groups are almost identical in all factors (covariates) that might impact the outcome of the experiment. For example, if we are comparing two treatments for lowering blood pressure, we want to make sure the two groups have roughly the same age distribution, to avoid concluding one is better than the other just because it was given to younger people.
The real goal of randomisation, though, is not to achieve perfect balance. To do that, one can use different methods built to specifically balance certain factors. For example, if we want to balance on age, and we already recruited the whole group of participants in our study, then we could split them into the two groups that would best achieve same age distribution. Looking back at the league table example, this is exactly the difference between the two designs we proposed: in one (European) we balanced precisely the matches in terms of opponents and home/away matches. In the other one (American) we didn't do that, so that we likely never ended up with a perfectly balanced design. Yet, the probability of facing each other opponent, and of playing the next game home or away, was always exactly the same, so the design, though slighlty less efficient, was still fair. Furthermore, differences between the two designs were not that large.
One might now think: even though the difference was small, why shouldn't we always choose the more efficient design? In the design of league tables this might be a sensible objection, but not in the design of randomised trials. There are several reasons why, but let's focus on one: randomised trials are like leagues where you do not know at the start of the season which teams will participate. Recruitment into trials is generally gradual, so you do not know at the design stage who the participants will be. Furthermore, even if you did, which is unlikely in clinical trials, but might be possible in other settings, you might not know all the factors you should balance for. It might not be just age, but sex as well. And what if there is a gene that has a big effect on the outcome, and that nobody knows about? What if, by balancing on known factors, we systematically created an imbalance for unknown ones? Because of this, the goal of randomisation in a clinical trial is not to balance perfectly, but to leave residual imbalances to chance alone. Broadly speaking, this guarantees that the groups receiving different treatments are exchangeable. The fact that usually they are also quite well balanced is just a nice additional characteristic of the design. Simply put, if we tossed a fair coin 500 times, we would have very low probability of getting exactly 250 tails and heads. But the important thing would not be to get exactly 250 each, but that each toss had the same probability of getting head or tail. Going back to our league example, when you do not know which teams will play in your league, what fairer strategy than drawing each matchday calendar completely at random?
I've got the Power
Thus far, we have talked about design mainly in terms of choosing a structure for our experiment. However, it is also important to decide how to size our experiment. In the American-style league example, we assumed each team would play precisely 30 games. However, there was no reason to choose that specific number, apart from making the comparison with the European-style more like-for-like. A possible strategy to choose a specific size for our experiment (aka sample size) is to target a specific performance measure. Let's say for example our goal is to have a design that, under our prior assumptions on the strength of the various teams, lets the best team win around 70% of the times. What's the percentage of leagues won by the strongest team when playing, say, 10 to 80 games?

Playing 10 games only, is enough to declare the strongest teams the league winner only around 40% of the times. This proportion increases gradually the more matches the season involves, until it reaches approximately our desired value for n=80: with 80 matchdays, this design would lead to the best team winning 70% of the times.
This is a concept very similar to one used to design randomised trials: power. What we want to do in a trial is to investigate whether a treatment works. So what we do while designing is decide what is a reasonable effect that would be considered important (e.g. a difference of 20% more people recovering within 2 weeks) and then find for which sample size (for which number of patients) we could find that the treatment works under that assumption 80%, or 90% of the times.
It is important to stress that this is dependent on the assumption one makes: the sample size with 80% power to show a treatment "works" if the difference is 20%, will not be enough to achieve the same power if the true difference is 10%. Similarly, in our league example, we made some very strong assumptions on the strength of all the teams. If we changed these, for example assuming there was a team much stronger than the opponents, let's say a Celtic, which would win 80% of the home games and 60% of the away games even against the second strongest team, our power to let it win would reach 70% even with as little as 5 or 6 games!

Cups and underdogs
The difference between the two league systems with the same sample size is quite small. Why is that? The reason is that, under the assumptions we made over the strength of the different teams, the Regular Season design is not that bad. It gives to each team the same probability to face each opponent on each match day. So what would be a very bad design?
Talking in terms of fairness (probability that the best team wins), knock-out rounds are a terrible design. Let's compare three possible such designs: 1) 2-legs & seeding: one option is to have knock-out rounds based on home-away matches. It is possible to seed the teams, so that the 8 strongest do not face each other in the round-of-16. This is pretty much the design used in the main continental football cups, including Champions League (Europe) and Copa Libertadores (South America), although the former has a single final played on neutral ground. What proportion of cups are won by the strongest team using this design and under our original assumptions on strengths of the 16 teams? As little as 33.5%!
2) 2-legs, no seeding: Another option is not to have seedings, but to draw the matchings completely at random. Because of the higher number of first round matches between teams of more similar level, this design leads to a further loss of efficiency: only 30.6% of cups go to the strongest team!
3) 1-leg, no seeding: finally, instead of two matches, we could have a single match played on neutral ground, as it happens for example in the World Cup. This further reduces the proportion of wins by the strongest team to 25%.
So, while the difference between the two league types was tiny, cups seem to be much more subject to random variability. This on one hand goes against the principle that the best teams should always win, but on the other hand makes results more unpredictable and, according to some, "fun". Of course the more legs, the fairer this will become. For example, NBA play-offs are played on a best-of-seven format, which makes them much more reflective of teams' levels.
Analysis should match design An attentive reader might have spotted a possible problem with the comparison of league designs and cup designs: the analysis is different. While in the league scenario the analysis gives 3 points to winners, 1 for draws, and gives the title to the team bringing more points back home, in the cup winners of each match simply progress to the next round, even if they won at the penalty lottery. We said, though, that we wanted to compare different designs under the same analysis strategy.
Why is this important? First, for the sake of our exercise, if we didn't use the same analysis method it would not be possible to disentangle the effect of the design and of the analysis on the results. We would be left with the question: was it an inferior design or an inferior analysis? Anyway, for this example, this may not be too important, as generally the teams who progress will make more points than the opponents. In the 1-leg scenario this will always be the case, while there are few cases in the 2-leg scenario where a team might win despite making virtually less points than the other finalist. But this would be rare.
However, the most important thing is that the design needs to assume the correct analysis model. And, similarly, the analysis needs to take the design correctly into account. Afterall, if you put a certain locker at home, you'll need the correct key to open it without having to break in.
Imagine for example that, after having designed our American-style league assuming the standard point system (3-1-0), we decided to analyse it differently. For example we decided to give 1.01, or 1000 points to match winners instead of 3. The first is an example of an analysis model that gives pretty much the same importance to draws and wins, but hugely penalises losses. The second is the example of a system that hugely values wins, at the expense of draws.
Because of this, we expect the first system not to reach the power targeted at the design stage (53%) and the second to possibly exceed it. This is, in fact, what happens:
1.01 points per win: 49%
3 points per win: 53%
1000 points per win: 55%
So, using a different analysis model than the one assumed at the design stage can both lead to a larger or smaller probability of success (power). Of course one could argue that exceeding power is not such a bad thing. That is generally true, in a world where resources to conduct our experiments were endless. This is often not the case, though; power larger than desired also means we could have reached the desired level with less matches.
When the only consequence of this error is to have a bunch of games more, this might not seem that much of a problem, but think about it in the context of a randomsied trial: this might mean exposing more patients than needed to an experimental treatment, taking way more time to get an answer and spending way more money.
OK, that "12 MIN READ" banner at the top is always quite intimidating, so I'll stop here. Did I say everything about design of statistical experiments? Hell, no. Did I at least provide the basic concepts? Probably still no. I could have talked more about exchangeability, about how the design is important particularly to answer causal questions, about how it is also important for observational studies... All things I might discuss in future posts. What I hope I manage to communicate with this post, is how the design is at least as important as the analysis for the success of a statistical experiment. In the end, probably none of this was necessary to tell this. A joke about a can and a lid would have been enough.