Popper, Arbuthnot, human sex ratio and statistical tests.
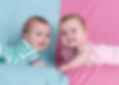
Taking decisions is the meat of life. We all know how difficult that sometimes can be, no matter whether the choice to make relates to our future career, our love life, or the cereals to buy for breakfast. Often, we dream of having our fool proof strategy to make decisions. But we know this is at best a naive hope.
Taking decisions in science is no different. Of course, though, any decision taken can have a much bigger impact than the choice between Weetabix and Corn Flakes, and hence even stricter rules must apply. In general terms, the set of rules used to advance scientific knowledge is called the scientific method.

The Scientific Method The father of the modern scientific method (and possibly of modern science itself) is considered by most to be Galileo Galilei. His method requires one should (i) start from experience, (ii) make some hypothesis and (iii) go back to experience, looking for confirmation (or rejection) of the formulated hypothesis using data from experiments. Mathematics and real life experiments were equally important to him. But what type of experiments should we make to advance our knowledge? There are broadly two possible types of experiments: those to verify that a hypothesis is correct, and those to prove that it is wrong, i.e. to falsify it. Modern science is vastly based on the second type of experiments, relying on what Karl Popper defined as the concept of falsifiability.
Imagine we were the first humans on earth. For few days we observe that daylight lasts for approximately 12 hours (yes, we are the first humas on earth but we do have clocks, don't ask me why). That happens for one, two, three days. We are tempted to conclude that daylight on earth always lasts 12 hours. However, for science, this is not enough. There are two ways to check whether our assertion is true: the first is to measure precisely daylight hours in every single point on earth every single day for eternity...

Alternatively, we assume that our statement is true unless we can find a single point in space (or time) where that is not the case. Clearly, the first approach is unfeasible for a variety of obvious reasons, while with the second approach it would be possible to make a simple experiment to prove our hypothesis wrong. For example, it would be enough to go to one of the Poles, or alternatively to travel north for a thousand kms or so and to wait for a few days. One such experiment would be enough to prove that daylight can last varying amount of times on earth. Our hypothesis was then falsifiable.
Not everything is falsifiable. The existence of God, for example, is largely unfalsifiable: no experiment could prove that God does, or does not, exist, and hence it is scientifically pointless to try and prove that. It is just a matter of one's beliefs. The main character of today's story, though, lived 200 years before Popper, and so he thought otherwise...
A 17th century maverick
John Arbuthnot was a Scottish physician, for few years the personal doctor of Queen Anne (The severely sick British Queen depicted by Olivia Colman in Lanthimos' movie The Favourite). From all the stories I read about him, I can clearly figure what kind of guy he was: full of humor, never trivial, apparently naive... in a word: a maverick. As most geniuses from the past, his talent led him to excel in several fields. Hence he also became famous as a satirist and as a mathematician. It is wearing his mathematician hat that he reportedly proved the existence of God, or at least its interference with this world.
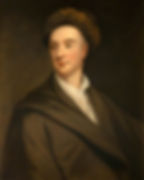
Arbuthnot, following the scientific method, started from experience to formulate a hypothesis: chance decides gender at birth. He then went on to collect data to perform an experiment to reject this hypothesis. In particular, he gathered data on christenings that had been collected in London for almost a century, and compared the number of boys and girls christened each year. Surprisingly, for 82 consecutive years, more boys than girls had been christened. What was the probability of that happening by chance alone?
Before looking at his answer to this question, we must ask ourselves another question: what did by chance mean to Arbuthnot?
Chance vs What?
In one of my previous posts I talked about binary random variables following a Bernoulli distribution; we generally use them when we want to model an event subject to chance that can take two values only. In this case, birthsex can either be boy or girl, so we can consider it to be the realisation of such a Bernoulli random variable. However, remember we said a Bernoulli probability distribution has a specific parameter : this is a number that we need to quantify the probability that the variable takes one of the two possible values. Let's call this p, the probability that the baby is a girl.
Arbuthnot lived in a completely different era; he had no idea of what a Bernoulli distribution was, but, in modern terms, his concept of chance was simply such a distribution, with p equal to 0.5, or 50%. Chance meant to him that the two events were equally likely; birthsex could have been decided with a coin flip. There was no space in his definition for different values of p. His coin was necessarily a fair one.
Now let's go back to the hypothesis that he wanted to falsify: chance decides babies' birthsex. Translated in modern statistics terms, he wanted to falsify the claim that a baby's birthsex follows a Bernoulli distribution with p=0.5. He proceeded in his experiment calculating the probability that, if what he wanted to falsify was indeed true, there would have been more boys than girls for 82 years in a row. The probability that more boys come to life on the first year is 50%, or 0.5. For two consecutive years, it is 0.5 multiplied by 0.5, 0.25 or 25%. For three years it is 50% of 25% and so on and so forth... To cut it short, he calculated the probability to have more baby boys than girls for 82 years in a row to be equal to 1 in (breathe deeply) 4 836 000 000 000 000 000 000 000. Not even knowing how to read this number, he concluded that the probability was so small that his starting hypothesis had to be wrong. This was probably the first (or at least, one of the very first) statistical hypothesis tests ever carried out.
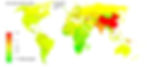
Statistical hypothesis tests
We said that, according to Popper, in order to reject a hypothesis it's enough to do a single experiment that finds it to be wrong. However, as usual, we live in a haphazard world. So, often we cannot conclusively say whether a statement is right or wrong, but just calculate the probability that, if it is correct, we'll see what we see. As the probability will never be zero, we then often choose a threshold below which we reject it. Most often than not, people use for this threshold the probability of 5%. If something that happened had less than 5% the probability of happening under a certain assumption, then we conclude that there is some indication that the assumption was likely not to be correct. Of course, using this approach, we broadly end up wrongly rejecting our assumption 5 out of 100 times even when it was correct.
If we wanted to be even more conservative, we could choose a smaller and smaller value as our threshold. This is not the main topic I'd like to discuss today, but some statisticians believe that it is instead simply wrong to choose any threshold, and that experiments should really just report the probability of the event happening under our original hypothesis, generally referred to as the null hypothesis. All sort of information, and experience, available should then be used to decide whether there is sufficient evidence to reject our hypothesis. This is one of the sources of huge discussions among statisticians, and not one that I could explain in few lines of a blog post.
The important message here is that calculating the probability that some veents happen under the assumption that a null hypothesis is correct, and using this probability to accept or reject it, is what is known as statistical hypothesis testing and, to my knowledge, Arbuthnot was the first in history to use this systematically. As usual, others before him might have used similar reasoning, but this would not take away any gram of importance to his experiment.
The Divine Providence
But let's go back to Arbuthnot: in his case, the probability of observign such extreme values that he calculated was so low that possibly everybody would have concluded that hypothesis was probably wrong. However, here he made a big bistake. Ironically, him being maybe the first man to ever do a statistical hypothesis test, he made the very same mistake that thousands of people were to do for decades after him: he did not think carefully about the alternative hypothesis. When we calculate the probability to observe what we observe in our experiment if our null hypothesis is true, we have to consider that the probability relies on all of the assumptions that are included in our null hypothesis. We cannot just focus on the ones we care about.
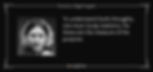
Arbuthnot, in modern terms, was testing whether the distribution of sexes was following a binomial distribution with p=0.5. Given the very low probability of observing more baby boys being christened for 82 years in a row if his null hypothesis was true, he claimed this to be probably not correct, and he concluded that the alternative hypothesis had to be considered more likely. Today, we'd say this alternative is that the probability p that a baby is a girl is not 0.5, or 50%. In fact, it is nowadays well known that on average, around the world around 48-49% of babies only are girls. The reason for this is not clear. Somebody believes it is Darwin's natural selection at work: since women live longer on average, to mantain approximately equal number of people from both genders, Nature might have made it more likely for a baby to be a boy at birth. Some others believe there must be a genetical reason, which would agree with the fact that the ratio is different across different ethnicities. For example, in China, up to 54% babies born in recent years happened to be boys. In some cultures, it is likely that differences are sadly due to sex-selective abortions, but overall the ratio is slighlty biased in favor of boys all over the world.
Arbuthnot alternative hypothesis, though, was not that p was different from 0.5. It was a much more curious one: if it is not chance (p=0.5), then it must be "Art" that governs. Translated, Divine Providence had regulated the birthsex ratio, and hence in his mind he had proven the existence of God, or at least its interference with our world.
While this might seem funny, or disingenous at best, to somebody living in our age, we must remember that everybody lives in their own time. Arbuthnot made his research before Popper discussed falsifiability; he applied statistical methods before statistics was fully developed as the discipline that we know since the 20th century. Still nowadays, decades after these methods have been developed, people keep claiming to have proved creative alternative hypotheses, just by showing the results of dubious experiments to disprove irrealistic null hypotheses. It is the same mistake, but a much less justifiable one.
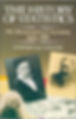
PS: I learnt about the story of Arbuthnot in the fantastic book "The History of Statistics" from one of the (if not The) most important statistical historians in the world, Stephen Stigler. If you like statistics and history, and you are not scared about a much more technical approach to the matter than the one that I take in this blog, I very much recommend it!